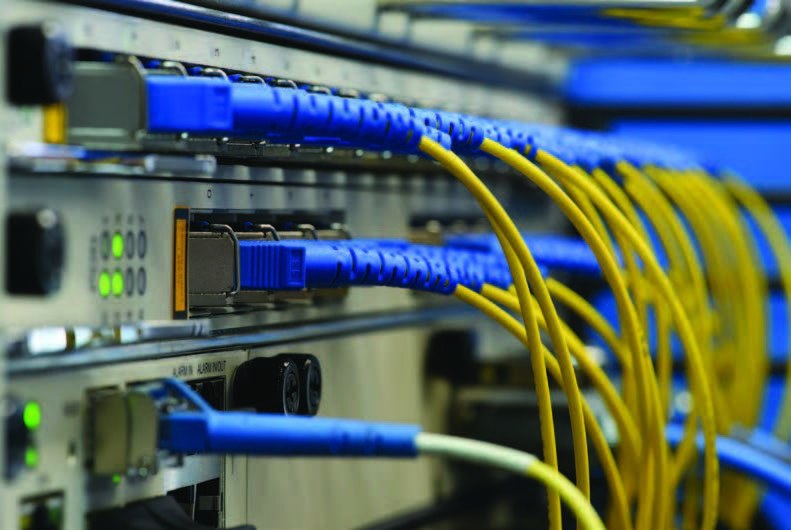
CASE STUDY: Dynamic optical network End-to-End QoT prediction
QoT (Quality of Transmission) prediction was deployed over a 986-km field trial using NDFF. Two cascaded ANN-based link penalty models in a dynamic optical network were developed for the QoT prediction and the prediction precision is up to 99.96% for R-square score.
Quality of transmission prediction has recently attracted increasing interest among researchers. Analytical model-based QoT estimation provides system-level optimization for static operations. In low-margin dynamic optical networks, precise QoTs should be predicted based on traffic payload, link configurations, and other parameters with further reduced link margins. In this work, two cascaded multi-channel ANN models are developed, co-trained, and demonstrated to predict link penalties over a 986-km field-trial NDFF testbed consisting of two links and ROADMs.
Fig. 1 Testbed setup of the 986-km field-trial
Figure 1 presents the 986-km field trial setup, which includes three nodes, and two optical links over part of the UK National Dark Fibre Facility (NDFF) that connects multiple sites with commercially available fibres and equipment. By configuring the optical switch, optical signals dropped at different nodes will be sent to the corresponding transponder for BER measurements, which are converted to Q-factors during data processing. With this configuration, 1306 data samples with different channel payloads at both links and Q-factors measured at three nodes have been collected from the field trial.
Fig.2 Results of the trained ANN-based penalty models
Figure 2 depicts the results of the ANN2 model for the second link. The learning curves of the ANN2 model training with different datasets, i.e., individual training with full measurements and co-training with inferences of ANN1 are presented in Fig. 2(a). Fig. 2(b) shows the comparison of the prediction Q-factors with the measurements of the two ANN models for both links. With the two ANN models for the two links, the end-to-end performance for optical channels can be predicted by cascading the two models, as shown in Fig. 2(c).
The prediction error of end-to-end is presented in Fig. 2(d), which shows that all the prediction errors are within ±0.16 dB. The error metrics of the two ANN models and the cascaded models are summarized in Table 1. It can be seen that the implemented ANN models perform with a low mean square error (MSE) of around 0.005 and mean absolute error (MAE) of around 0.045. The high prediction accuracy of up to 99% of the R-square score (R2) validates the feasibility of ANN model cascading and the capability to be scaled up.
In summary, NDFF is proven to be an experimental facility for our system to allow for dynamic optical network monitoring, data collection and dynamic configuration with three-node two-link real dynamic optical network. Cascaded ANN-based traffic-aware link penalty prediction models are proposed and demonstrated over the 986 km NDFF testbed to provide end-to-end QoT predictions with a prediction error within 0.16 dB. The proposed co-training methods use the trained penalty models for previous links to provide the required data that is impractical to obtain in practical networks for the following link, providing a possible way to train all link penalty models in a complex network topology. By cascading the link penalty models, network-level QoT prediction can be achieved.
Funding information: This work has been funded by European Commission's Horizon 2020 research and innovation program under grant agreement No 871428, 5G-CLARITY project.
For further information: contact Prof. Dimitra Simeonidou, ndff@ee.ucl.ac.uk
Published: 10 March 2023